The Executive Guide to Artificial Intelligence Summary
12 min read ⌚
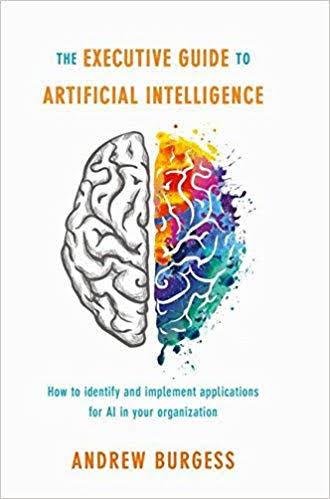
How to Identify and Implement Applications for AI in Your Organization
Sure you know what AI is, but do you know how to implement it in your organization?
If not, let Andrew Burgess be your guide:
The Executive Guide to Artificial Intelligence.
Who Should Read “The Executive Guide to Artificial Intelligence”? And Why?
To quote a pinned March 24, 2018 tweet by the author, The Executive Guide to Artificial Intelligence is “for anyone who is thinking of implementing AI in their organization and needs to know where to start.”
Since, in addition to a strategy to begin your AI journey, it also explains AI in non-technical terms, most of the book (with the exception of the last two or three chapters) is suitable for anyone who wants a comprehensive introduction to AI.
At less than 200 pages, you can’t get much better than the one Anthony Burgess provides here.
About Andrew Burgess
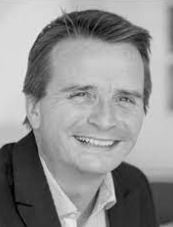
Andrew Burgess is an English author, most renowned as a strategic advisor on Artificial Intelligence, RPA, and innovation.
Considered an authority on innovative and disruptive technology, Burgess has worked as a management consultant, author, and speaker for over 25 years.
A former CTO, he is currently a member of the Advisory Boards of numerous ambitious disruptive companies and is involved in many major change projects (strategic development, IT transformation, outsourcing) in a wide range of industries.
The Executive Guide to Artificial Intelligence is the first book where he appears as the only author.
“The Executive Guide to Artificial Intelligence Summary”
Whether you are a fan or not, AI is one of the most talked-about technologies in the business world today.
How can it not be?
It delivers numerous benefits as it is, and it promises to deliver even more as it is being perfected.
The problem, however—as it is often the case when new technologies emerge—is that very few executives and managers understand what AI offers and even fewer are willing to investigate the matter, repelled and confused by the “bewildering bundle of algorithms and jargon,” by the “confusing terminology, inflated expectations and dire warnings of ‘robot overlords.’”
Even though the truth is not at all simple—suggests Andrew Burgess in The Executive Guide to Artificial Intelligence—it can be simplified.
Hence, his book, whose mission is not only to demystify AI for everyone interested in it but also to provide a blueprint for executives and managers who want to identify where it can help them and implement its applications in their organizations.
So, allow us to walk you through it!
Defining AI and Its Applications
Even though when most people think of AI think of the “robot overlords” mentioned above, AI is, in fact, everywhere around us.
Some of its uses today, remind us Burges, “as planning a route in our satnav or getting a phrase translated in our browser, are so ubiquitous that we forget that there is actually some really clever stuff happening in the background.”
Well, that clever stuff is done by AI, defined by the OED as “the theory and development of computer systems able to perform tasks normally requiring human intelligence.”
Now, that definition doesn’t tell the whole story, since debates are raging day in day out on whether AI should merely augment the human mind or replace it altogether. And they are said to be as old as the discipline itself.
According to a famous anecdote, one of the two “founders” of AI, Marvin Minsky, enthusiastic about the opportunities AI offers, told his colleague at MIT Douglas Engelbart that “we’re going to make machines intelligent. We are going to make them conscious!”
Engelbart, the first one to demonstrate “augmented human intellect” back in 1968 (in the “mother of all demos), supposedly replied: “You’re going to do all that for the machines? What are you going to do for the people?”
Well, Engelbart was a bit too pessimistic since AI can already do a number of things for the people who know how to use its potential.
For example, in addition to translating and navigating, It can also:
…read thousands of legal contracts in minutes and extract all the useful information out of them; it can identify cancerous tumors with greater accuracy than human radiologists; it can identify fraudulent credit card behavior before it happens; it can drive cars without drivers; it can run centers more efficiently than humans; it can predict when customers (and employees) are going to desert you and, most importantly, it can learn and evolve based on its own experiences.
No wonder the World Economic Forum is guiding us toward a reskilling revolution, and Yuval Noah Harari is warning millennials everywhere that when they grow up, they might not have a job.
That is how things stand even today: a robot might take your job any instant now (check the immediate risk here), and AI is to blame for that.
But that is not bad at all.
A Brief History of AI
Since we already mentioned Minsky and Engelbart, it is only appropriate that we mention a thing or two about some other historical developments in the field of AI so that we can understand how we got to be on the brink of automation.
In a nutshell, the evolution of AI is the development of controlled if/then workflows through guided learning systems to machine learning.
As mentioned, in the beginning, AI comprised nothing more than the development of “if this than that” programs called “expert systems.” These expert systems were basically knowledge maps, branching out in one or another direction based on the choice made by a user.
Under the guise of AI, many of these systems are still in use today: most of those chatbots you encounter on your favorite websites use nothing more than rudimentary AI.
Due to little funding and over-inflated expectations, AI passed through two “AI Winters,” during which advances in the technology stagnated. Both of them lasted for six years (the first one between 1974 to 1980, and the second one from 1987 to 1993), but nowadays it is very different due to “four key drivers”:
• Big Data: AI needs “millions of examples” to be accurate, but today’s continual use of the Internet provides it with even more data than necessary;
• Cheap Storage: In 1980, 1GB of storage cost, on average, $437,500. Just a decade later, it was a fortieth of that price: $11,200. Nowadays, 1GB of storage costs less than 2 cents!
• Faster Processors: we’ve already told you a little bit about Moore’s Law; well, it turns out its predictions were accurate—processors are getting smaller and faster every single year.
• Ubiquitous Connectivity: up until 1993, very few people had Internet; now, very few people don’t. That’s a big difference.
Thanks to these key drivers, AI is getting better by the second and is now able to learn in an unsupervised manner.
In the more common supervised learning, AI systems are trained and guided by other people. For example, if you want AI to learn what is a dog, you can train it by “feeding” it with millions of images and telling it which ones of them are dogs, and which are not.
However, in the more SF unsupervised learning, systems start with a blank slate, and they are fed data which initially means nothing to them. In time, however, they are able to notice patterns and make sense of it.
AI Capabilities Framework
Andrew Burgess has developed a framework that “boils down” all of AI’s complexity into eight capabilities, subsequently divided into two sets:
• Image recognition
• Speech recognition
• Search
• Clustering
• Natural Language Understanding (NLU)
• Optimization
• Prediction
• Understanding
Now the first four capabilities listed above (image and speech recognition, search, and clustering) focus on figuring out “what is happening” in a data set. They identify, distinguish and group things, converting unstructured data to structured data.
The second set deals more with using the structured data (“why is it happening”) in one way or another and, unlike the first set (which is training-based) includes some type of machine-based (almost independent) output.
NLU, for example, includes a degree of understanding and it requires cognition. It is through a similar type of cognition that machines are able to transform data from one form or another (optimization) or even predict whether something will happen or not (prediction).
The last of these eight capabilities—understanding—is still not commercially available, but it is what most people expect AI to become: conscious awareness of a machine of its thoughts and actions.
AI in Action
Though Artificial Intelligence can do a lot of things, it can’t do everything. But in combination with some associated technologies (cloud computing, robotics, IoT, or crowdsourcing), it can change absolutely everything about the world that we know.
In fact, it is already changing things!
We’ve already mentioned chatbots which are so simple that they are not even considered AI anymore. But they do do things for which human intelligence is necessary and that is a great thing. Complex chatbots can do so much more: through extensive training, they can become almost indistinguishable from humans—already.
All of the recommendations you get on Amazon or Netflix are due to AI, which is immensely better than humans in suggesting future purchases (optimization & prediction) based on previous acquisitions (search & clustering).
British retailer Tesco combines AI and robotics to ease restocking. Through image recognition, robots identify product gaps by filming the shelves and comparing them to the shelves’ optimal condition.
Possibly even more interesting and less known, an Israeli tech company named Nexar teaches people to become better drivers by using and structuring information from a dashcam app.
All in all, the options are many, and leaders should identify the spheres where AI can help them and act as soon as possible.
Your AI Journey
Burgess is often asked by executives how can they implement AI in their business. He claims it is the wrong question. The much more appropriate one is: “I have some big business objectives/challenges—how can AI help me deliver or address them?”
Well, this is how.
The “AI Maturity Matrix”
As is the case with everything else, implementing AI starts with self-assessment, and Burgess suggests using the “AI Maturity Matrix” before doing anything else.
Originally developed by Carnegie Mellon University to assess the maturity of IT development functions, maturity matrices generally describe five levels of maturity—from very immature (Level 1, or ‘Initial’) to world-class (Level 5, or ‘Optimizing’).
However, the “AI Maturity Matrix” has six levels rather than five:
Level 0: Manual processing. This is the basic level when there is very little evidence of any IT optimization in an organization.
Level 1: Traditional IT-enabled automation. The organization has implemented task-specific IT applications for particular processes (e.g., an invoice processing application to process invoices), but has not applied automation more broadly.
Level 2: Isolated, basic automation attempts. Some people in an organization do things automatically (using scripts or macros) with little to no benefit; data is still managed on a nominal basis.
Level 3: Tactical deployment of individual automation tools. At level 3, an organization starts tactically applying a single automation tool to meet specific goals.
Level 4: Tactical deployment of a range of automation tools. At this stage, an organization already has a reputation for implementing new technologies and being innovative, since it has started deploying a range of automation tools, whose benefits are observable and sizeable.
Level 5: End-to-end strategic automation. At this level, an organization has implemented a strategic end-to-end process automation program with a range of automation tools, including AI.
AI Heat Map
In addition to this “AI Maturity Matrix,” Burgess advises creating an “AI Heat Map” as well so that a company can get “a top-down perspective on areas where AI is desirable, economically viable and/or technically feasible.”
Industrializing AI: Musts and Hazards
There are many steps between the “AI Maturity Matrix”/”AI Heat Map” and the implementation of AI in your organization—say, developing the AI business case, an AI roadmap, an AI strategy—but let’s say you’re there. What’s next?
Well, first of all, you must align everything with your new vision: a Level-5 AI-based automated company. This includes adding new positions such as CAO (chief automation officer) and CDO (chief data officer).
The last one is especially important if you take into consideration that with AI, data is everything, and “data fidelity” can make or break AI.
Speaking of which, there’s always the hazard that AI can also break your company, since, much like Sheldon from The Big Bang Theory, it doesn’t understand social conventions, and can be hacked, via training, by people with malicious intentions. (Just ask Microsoft’s Twitter bot Tay who became a racist after a single day).
Finally, AI can be used as a tool against AI. For example, if you are a bank, and you implement voice recognition as part of your safety system, an AI can be trained to mimic the voices necessary to gain access to a specific account.
The Future of AI
Be that as it may, AI’s future looks brighter than ever!
Image and voice recognition have been improving so well during the past few years that, very soon, it will be difficult to recognize whether you’re talking to a bot or a real person on the phone (not to mention, impossible to distinguish fake from real news).
Clustering and searching are becoming ever more efficient and accurate, which means that optimization and predicting will also improve. The only problem—and it will be a problem for a while—is the lack of high-quality data sets, but Burgess believes that will find a solution for that as well. Afterward, both NLU and Understanding will improve to almost SF-extents.
If you don’t want to be the last to hop on the bandwagon, you better do it right away!
Key Lessons from “The Executive Guide to Artificial Intelligence”
1. AI Is Everywhere Around You
2. AI’s Complexity Can Be Boiled Down into a Framework of Eight Capabilities
3. AI Is Here to Stay—and You Better Implement It in Your Organization
AI Is Everywhere Around You
Whether you’re using Google Translate to understand what the tattoo of your favorite pop artist means in your language or you’re navigating through the streets of Cairo, whether you are searching for very specific information in a large bulk of data or simply clicking on images of traffic lights in captchas—you are actually either using or training AI systems.
AI’s Complexity Can Be Boiled Down into a Framework of Eight Capabilities
Even though AI is a complex phenomenon, Andrew Burgess has devised a framework that boils down its complexity into eight capabilities: image recognition, speech recognition, search, clustering, natural language understanding (NLU), optimization, prediction, and understanding.
The first four of these skills help AI understand “what is happening” in a given data set, and in the other four it is coming up with a solution as to “why it is happening.”
Almost all AI-based apps can be explained through the use of these eight capabilities.
AI Is Here to Stay—and You Better Implement It in Your Company Fast
Every time you search for something on Google or post something on Facebook, you are feeding AI—which makes it stronger and better. At least for now, there’s no way to stop this, meaning you better join in.
No matter what kind of an organization you lead, AI can help you in numerous ways, so you better assess how mature you are for the transformation (via an “AI maturity matrix”), identify how can AI help you improve (via an “AI heat map”) and start implementing it and automating processes!
Like this summary? We’d like to invite you to download our free 12 min app for more amazing summaries and audiobooks.
“The Executive Guide to Artificial Intelligence Quotes”
Artificial intelligence is being used in businesses today to augment, improve and change the way that they work. Click To Tweet It’s impossible to get value out of something if it is not understood, unless it’s by some happy accident. In the world of AI, there are no happy accidents; everything is designed with meticulous detail with specific goals in mind. Click To Tweet The first driver for the explosion of interest and activity in AI is the sheer volume of data that is now available. Click To Tweet The reason machine learning is called machine learning is, rather obviously, that it is the machine or computer, that does the learning. Click To Tweet If there is trust and transparency around the data that consumers find useful, then they are more likely to allow businesses open access to that, therefore increasing the utility even further. Click To TweetOur Critical Review
Highly accessible even to a novice, Andrew Burgess’ book is not only recommendable to anyone with even a vague interest in modern technology but also essential for everyone who wants to implement AI in his company.
Even though quite brief and not proofread as a book of its kind is supposed to be, The Executive Guide to Artificial Intelligence certainly offers a lot and is precisely what the blurb states it is: a comprehensive introduction to AI and everything related, especially suitable for managers and executives.
We dare you to not feel a bit smarter after finishing the book.
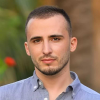
Emir is the Head of Marketing at 12min. In his spare time, he loves to meditate and play soccer.